[論文介紹] PLAN-AND-ACT: Improving Planning of Agents for Long-Horizon Tasks
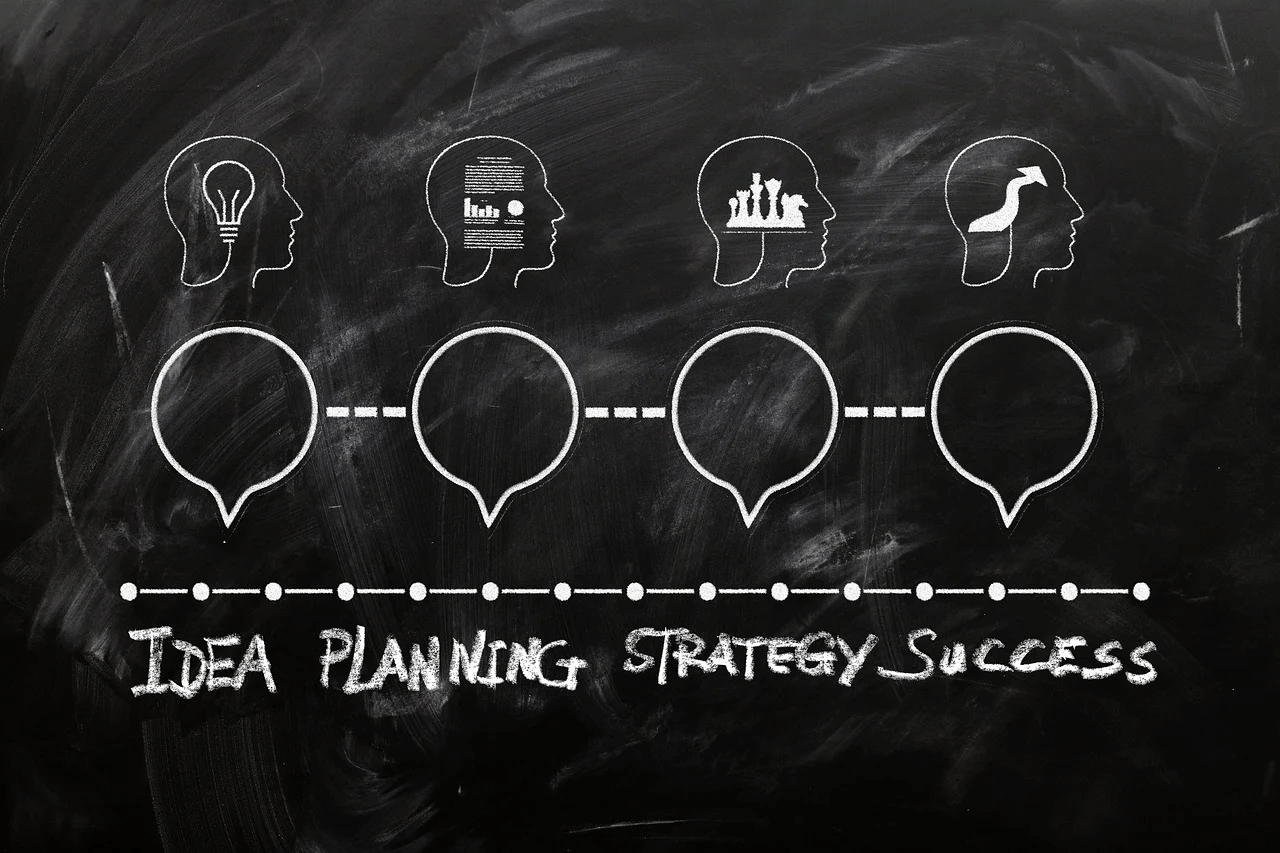
1 前言
本篇文章介紹 PLAN-AND-ACT: Improving Planning of Agents for Long-Horizon Tasks 論文,PLAN-AND-ACT 主要由 UC Berkeley 的研究人員提出,並於 2025 年 3 月發表於 Arxiv。
PLAN-AND-ACT 提出一個 Planner-Executor 框架以及資料合成方法來訓練 LLM,提昇 LLM 的規劃 (Planning) 以及執行 (Execution) 能力。
如下圖所示,PLAN-AND-ACT 主要由 Planner 與 Executor 組成。由 Planner 根據使用者給的任務先產生「計畫」,所謂的「計畫」其實就是一連串較為高層次的目標,再由 Executor 根據這個計畫轉為環境中特定的行為。
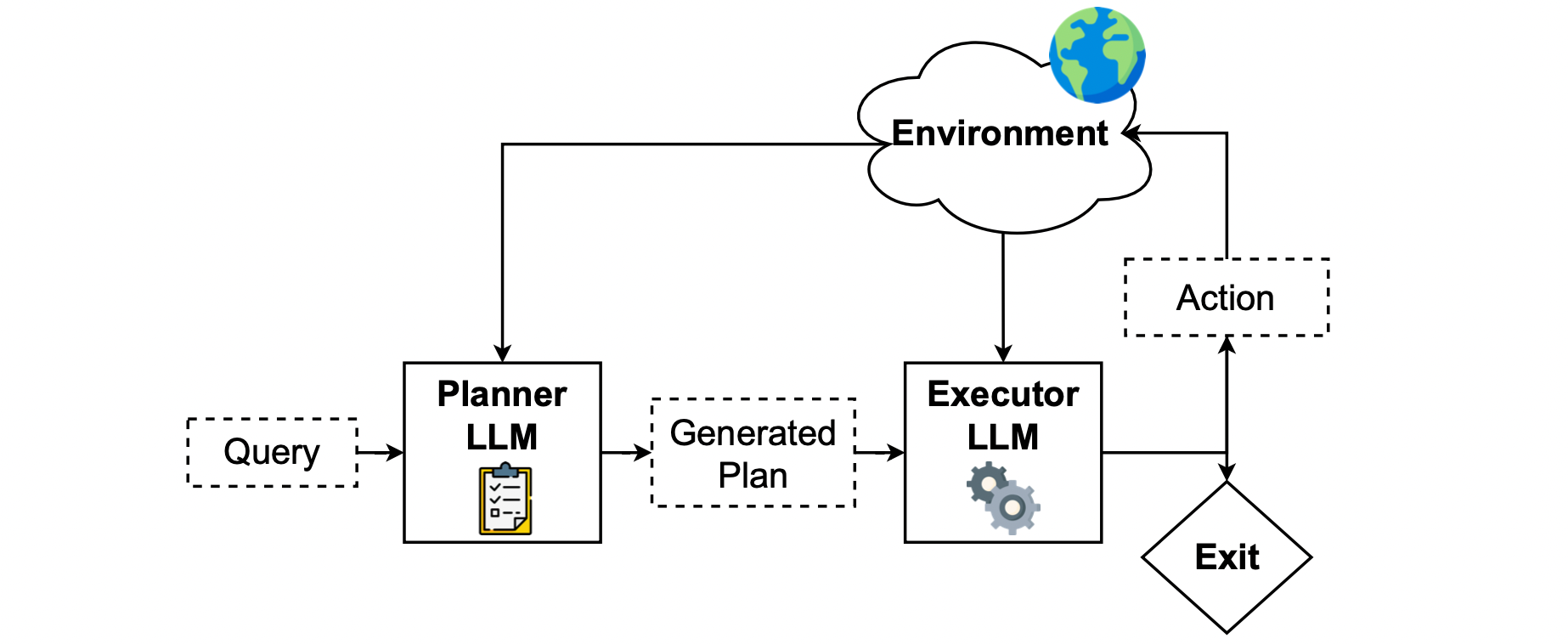
2 PLAN-AND-ACT 想解決的問題
PLAN-AND-ACT 想處理的問題正是 LLM 的規劃 (Planning) 能力。現有的 LLM 在 Planning 上具有以下挑戰:
- LLM 通常難以將使用者所提供的 High-Level 目標轉為具體的 Plan(例如:「幫我訂一張飛往紐約的機票」)分解為具體且可執行的步驟(例如:「打開航空公司網站」、「輸入旅行日期」等)
- 即使 LLM 可以產生 Plan,但隨著任務變得更長且更複雜,Plan 中的步驟也會變多,導致 LLM 無法追蹤已經完成的步驟以及尚未完成的部分
- 即使 LLM 可以追蹤一個很長的 Plan,但現實生活中的環境通常是動態、隨機且不可預測的,LLM 很可能無法在一開始制定好 Plan,就按照這個 Plan 走到最後,而是必須動態的根據環境給的回饋來修改 Plan
- 回到 LLM 本質能力,由於缺乏 Planning 相關的高品質訓練數據,LLM 本身就不是被訓練成一個 Planner
3 PLAN-AND-ACT 提出的解決方法
為了解決上述關於 LLM Planning 的 4 個問題,PLAN-AND-ACT 提出 2 個解法:
- 針對 問題(1)-(3) 提出 PLAN-AND-ACT 這個框架,將 Planner 以及 Executor 分離,由 Planner 產生 Plan,由 Executor 負責執行 Plan
- 針對 問題(4) 提出一種在不管是否有 Groundtruth 下,都可以產生與 Planning 相關的 Synthetic Data 的 Pipeline,就能夠透過這些 Synthetic Data 訓練 Planner
4 PLAN-AND-ACT 框架
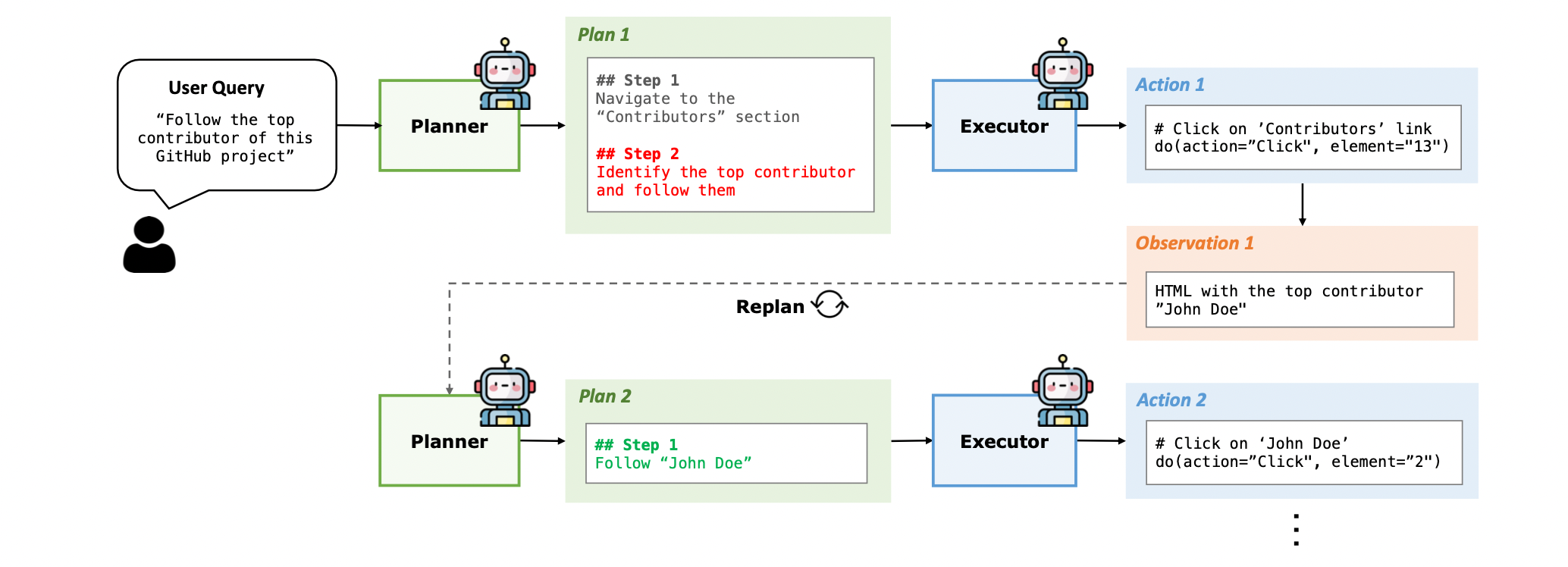
PLAN-AND-ACT 框架的核心在於避免透過單一模型同時處理 Planning 以及 Execution 任務,因此才有了 Planner 與 Executor 分工合作的架構。作者在論文中也明確提到:
PLAN-AND-ACT 框架理論上可以在任何環境中運作,作者在論文中以 Web (網際網路) 作為環境的例子。由於在 Web 環境中使用者給出的任務變化多端,很多任務也會需要很多步驟的規劃,因此作者認為 Web 環境很適合用來衡量 LLM 的 Planning 能力。
舉例來說,以上圖 Figure 2 而言,使用者可能詢問 “Follow the top contributor of this GitHub project”,這時候 Planner 可能會給出以下的 Plan:
- Navigate to the Contributors section
- Identify and follow the top contributor
Executor 在接收這個 Plan 後,就必須採取環境中相對應的行為,像是:
- 點擊特定的連結
- 在搜尋欄位中填入正確的內容
由於是在 Web 環境,Planner 以及 Executor 所得到的 Observation,都會是 HTML 格式的文字內容。
4.1 Planner Prompt
System Prompt
## Goal You are the Global Planner agent, an expert plan generator for web navigation tasks. You will be proivded with the following information: - **User Query**: The web task that you are required to generate a global plan for. - **Initial HTML State**: The initial HTML state of the web page. You are responsible for analyzing the usery query and the initial HTML state to generate a structured, step-by-step global plan that outlines the high-level steps to complete the user query. The global plan that you generate shouldn’t directly describe low-level web actions such as clicks or types (unless necessary for clarity) but outline the high-level steps that encapsulate one or more actions in the action trajectory, meaning each step in your plan will potentially require multiple actions to be completed. Your global plan will then be handed to an Executor agent which will perform low-level web actions on the webpage (click, type, hover, and more) to convert your global plan into a sequence of actions and complete the user query. ## Expected Output Format The global plan you generate should be structured in a numbered list format, starting with ’## Step 1’ and incrementing the step number for each subsequent step. Each step in the plan should be in this exact format: ‘‘‘ ## Step N Reasoning: [Your reasoning here] Step: [Your step here] ‘‘‘ Here is a breakdown of the components you need to include in each step of your global plan as well as their specific instructions: - **Reasoning**: In this section, you should explain your reasoning and thought process behind the step you are proposing. It should provide a high-level justification for why the actions in this step are grouped together and how they contribute to achieving the overall goal. Your reasoning should be based on the information available in the user query (and potentially on the initial HTML state) and should guide the Executor agent in understanding the strategic decision-making process behind your global plan. - **Step**: In this section, you should provide a concise description of the global step being undertaken. Your step should summarize one or more actions as a logical unit. It should be as specific and concentrated as possible. Your step should focus on the logical progression of the task instead of the actual low-level interactions, such as clicks or types. ## Guidelines: - Ensure every action and reasoning aligns with the user query, the webpage at hand, and the global plan, maintaining the strict order of actions. - Minimize the number of steps by clustering related actions into high-level, logical units. Each step should drive task completion and avoid unnecessary granularity or redundancy. Focus on logical progression instead of detailing low-level interactions, such as clicks or UI-specific elements. - Provide clear, specific instructions for each step, ensuring the executor has all the information needed without relying on assumed knowledge. For example, explicitly state, ’Input ’New York’ as the arrival city for the flights,’ instead of vague phrases like ’Input the arrival city.’ - You can potentially output steps that include conditional statements in natural language, such as ’If the search results exceed 100, refine the filters to narrow down the options.’ However, avoid overly complex or ambiguous instructions that could lead to misinterpretation. ## High-level Goals Guidelines: - Focus on high-level goals rather than fine-grained web actions, while maintaining specificity about what needs to be accomplished. Each step should represent a meaningful unit of work that may encompass multiple low-level actions (clicks, types, etc.) that serve a common purpose, but should still be precise about the intended outcome. For example, instead of having separate steps for clicking a search box, typing a query, and clicking search, combine these into a single high-level but specific step like "Search for X product in the search box". - Group related actions together that achieve a common sub-goal. Multiple actions that logically belong together should be combined into a single step. For example, multiple filter-related actions can be grouped into a single step like "Apply price range filters between $100-$200 and select 5-star rating". The key is to identify actions that work together to accomplish a specific objective while being explicit about the criteria and parameters involved. - Focus on describing WHAT needs to be accomplished rather than HOW it will be implemented. Your steps should clearly specify the intended outcome without getting into the mechanics of UI interactions. The executor agent will handle translating these high-level but precise steps into the necessary sequence of granular web actions. ## Initial HTML State Guidelines: - Use the initial HTML of the webpage as a reference to provide context for your plan. Since this is just the initial HTML, possibly only a few of the initial actions are going to be taken on this state and the subsequent ones are going to be taken on later states of the webpage; however, this initial HTML should help you ground the plan you are going to generate (both the reasoning behind individual steps and the overall plan) in the context of the webpage at hand. This initial HTML should also help you ground the task description and the trajectory of actions in the context of the webpage, making it easier to understand the task. - You MUST provide an observation of the initial HTML state in your reasoning for the first step of your global plan, including the elements, their properties, and their possible interactions. Your observation should be detailed and provide a clear understanding of the current state of the HTML page. ## Formatting Guidelines: - Start your response with the ’## Step 1’ header and follow the format provided in the examples. - Ensure that each step is clearly separated and labeled with the ’## Step N’ header, where N is the step number. - Include the ’Reasoning’ and ’Step’ sections in each step.
User Message
## User Query {user_query} ## Initial HTML State {initial_html_state} You MUST start with the ’## Step 1’ header and follow the format provided in the examples.
4.2 Executor Prompt
System Prompt
# Goal You are the Executor Agent, a powerful assistant can complete complex web navigation tasks by issuing web actions such as clicking, typing, selecting, and more. You will be provided with the following information: - **Task Instruction**: The web task that you are required to complete. - **Global Plan**: A high-level plan that guides you to complete the web tasks. - **Previous action trajectory**: A sequence of previous actions that you have taken in the past rounds. - **Current HTML**: The current HTML of the web page. Your goal is to use the Global Plan, the previous action trajectory, and the current observation to output the next immediate action to take in order to progress toward completing the given task. # Task Instruction: {intent} # Global Plan The Global Plan is a structured, step-by-step plan that provides you with a roadmap to complete the web task. Each step in the Global Plan (denoted as ’## Step X’ where X is the step number) contains a reasoning and a high-level action that you need to take. Since this Global Plan encapsulates the entire task flow, you should identify where you are in the plan by referring to the previous action trajectory and the current observation, and then decide on the next action to take. Here is the Global Plan for the your task: {global_plan}
4.3 Dynamic Planning
為了讓 Plan 可以更適應環境的變化,PLAN-AND-ACT 加入了 Dynamic Planning 環節。如同 Figure 2 所示,每當 Executor 執行了一個 Action 後,就會讓 Planner 依據原本的 Plan、Executor 的 Action 以及環境提供的 Observation 進行 Replan。
我覺得這樣的機制在 LLM Planning 的方法中算是直覺的做法,畢竟現實生活中的環境一定是多變的(例如:在網頁上突然跳出了一個新的廣告視窗)。如果 Planner 只產生一次 Plan 就讓 Executor 跟著 Plan 執行到最後,那麼很有可能會在過程中出錯。
在 PLAN-AND-ACT 中,Executor 每執行一次 Action,Planner 就會進行一次 Replan。這樣的作法雖然可以讓 Plan 與時俱進,但是也會帶來許多運算成本,大幅增加使用者得到回覆的時間。
5 LLM-Based Synthetic Data Generation Pipeline
如上方論文的論述,作者非常清楚直接地提及:單純靠 Prompting 來提升 LLM (Planner & Executor) 在 Web 環境的 Planning 以及 Executing 能力是遠遠不夠的。需要對 Planner 以及 Executor 進行 Finetune!
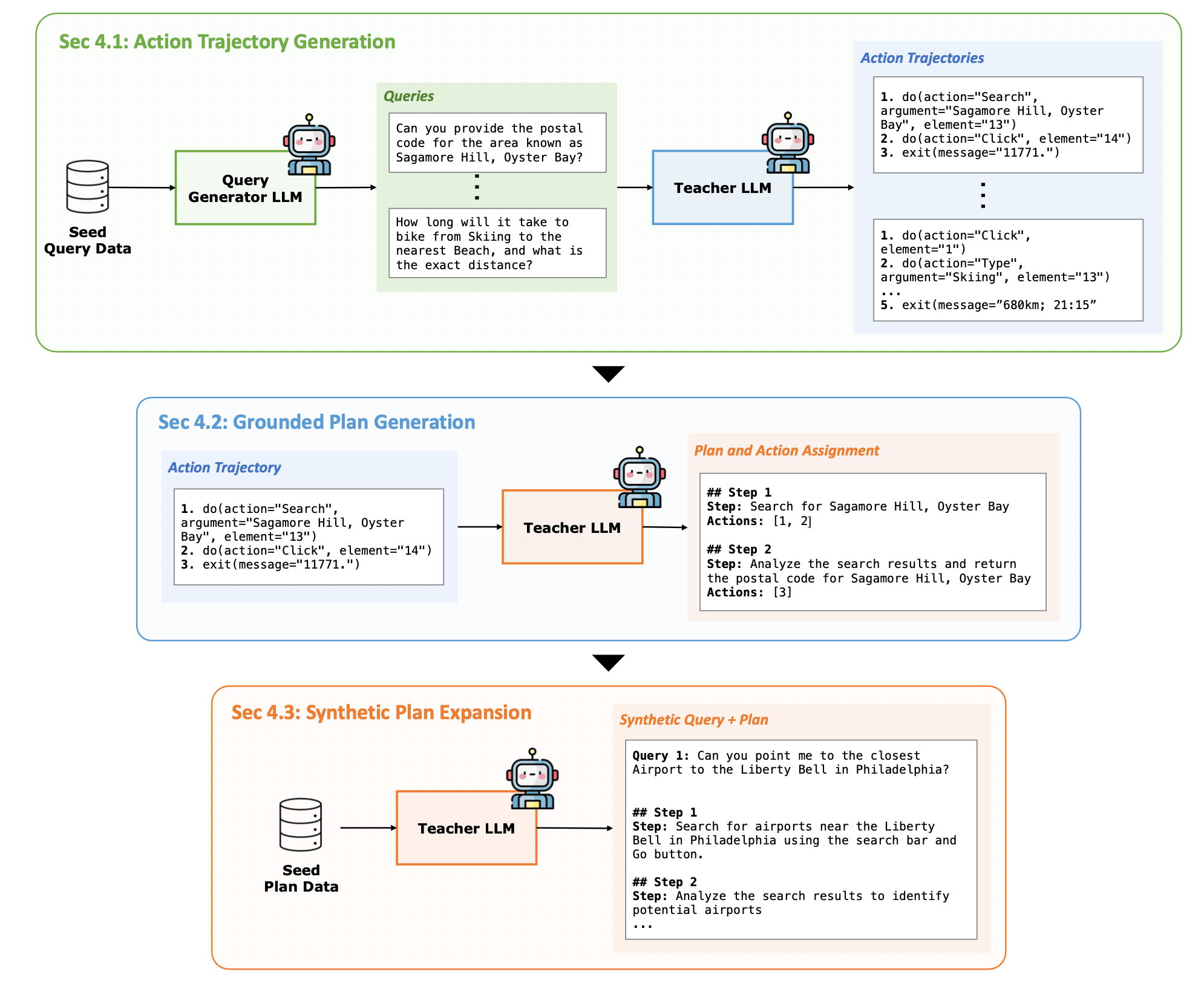
如上圖 Figure 3 所示,為了針對 Planner 進行 Finetune,作者提出了一個 Synthetic Data Generation 方法來生成 Planner 以及 Executor 的訓練資料。
5.1 生成 Executor 的訓練資料:Action Trajectory Generation
作者基於 WebRL (ICLR 2025 Poster) 的方法,隨機從訓練資料中 Sample 出一些 Query 作為 Seed Prompt,並利用 LLM 產生新的相似的 Query,再透過 LLM 將無法完成的 Query 過濾掉。
接著,這些新生成的 Query 會交給一個 Teacher LLM 嘗試,並將每一次嘗試的 Trajectory 收集起來。最後,再使用 Outcome-Supervised Reward Model(ORM)對這些 Trajectory 進行評分,來篩選出成功與失敗的 Trajectory。
透過這樣的流程,就能夠收集到許多成功解決任務的 Trajectory,就可以作為訓練 Executor 的訓練資料。
5.2 生成 Planner 的訓練資料:Grounded Plan Generation
要生成 Planner 的訓練資料的一個直覺作法:直接讓 Teacher LLM 根據使用者的 Query 來產生 Plan。
然而,這樣的作法明顯有缺陷。由於 Teacher LLM 並沒有真的接觸到環境,會導致其所生成的 Plan 與環境的提供的執行結果不對齊。此外,Teacher LLM 本身可能也沒有被特別預訓練在這樣的任務上,也會導致其所生成的 Plan 品質不好。
作者提出一個簡單的解決辦法,如 Figure 3 的第二列所示,作者 Prommpt Teacher LLM 成為 Reverse Engineer,將已經生成的 Execution Trajectory 轉為一個 Structured Plan。
為了讓產生的 Plan 可以真的對應到 Trajectory 中的 Action,Teacher LLM 被要求要將 Plan 中的每一個 Step 寫上其所對應的 Action。
5.3 生成更多的 Planner 的訓練資料:Synthetic Plan Expansion
Grounded Plan Generation 並不是一個有效率的方法:首先,需要先生成 Executor 的訓練資料 (Query-Trajectory Pair),再透過 Reverse-Engineering 將這些 Trajectory 轉為 Plan。
然而,問題在於在生成 Executor 的訓練資料的過程,Teacher LLM 經常會產生大量失敗的 Trajectory,這就影響到了最終可以使用的 Trajectory 的數量。
此外,針對一個成功的 Trajectory,如果裡頭包含了 8 個 Step,對於 Executor 而言相當於是 8 個訓練樣本,然而對於 Planner 而言,僅僅是 1 個訓練樣本 (只能代表 1 個 Plan)。
為了更大量的產生 Planner 的訓練資料,作者從已經生成的 Planner 的訓練資料中,隨機 Sample 出一些 Query-Plan Pair 作為 Seed Prompt,讓 GPT-4o 根據這些 Examples 來產生更多 Query-Plan Pairs。
5.4 提升 Planner 訓練資料的難度:Targeted Plan Augmentation
為了提升 Planner 訓練資料的難度,作者將一部份的訓練資料切出當作驗證資料,然後測試 Planner 在驗證資料上的表現。
針對 Planner 表現不好的失敗樣本,作者透過一個 LLM 來對訓練資料樣本進行分類,找到與這些失敗樣本相似的樣本。並將這些類似於失敗樣本的訓練樣本作為 Seed Prompt,讓 LLM 產生更多類似的樣本。
6 PLAN-AND-ACT 實驗
6.1 環境 (Benchmark) 選擇
- WebArena
- WebArena-Lite
- WebVoyager
6.2 模型選擇
- PLAN-AND-ACT 框架
- Planner 和 Executor: 基於 LLaMA-3.3-70B-Instruct 模型分別 Fintune 出 Planner 以及 Executor
- Dynamic Replanning: 基於 LLaMA-3.3-70B-Instruct 模型透過 LoRA Finetune
- Synthetic Data Generation Pipeline
- User Query Generator (in Action Trajectory Generation), Grounded Plan Generation, Synthetic Plan Expansion: 使用 GPT-4o
- Action Trajectory Generation: 使用 WebRL-Llama-3.1-70B 作為 Actor Model,並使用 ORM-Llama-3.1-8B Reward Model
- Planner 和 Executor 的 Chain of Thought Reasoning: 使用 DeepSeek-R1-Distill-Llama-70B 作為 Teacher LLM
6.3 實驗結果
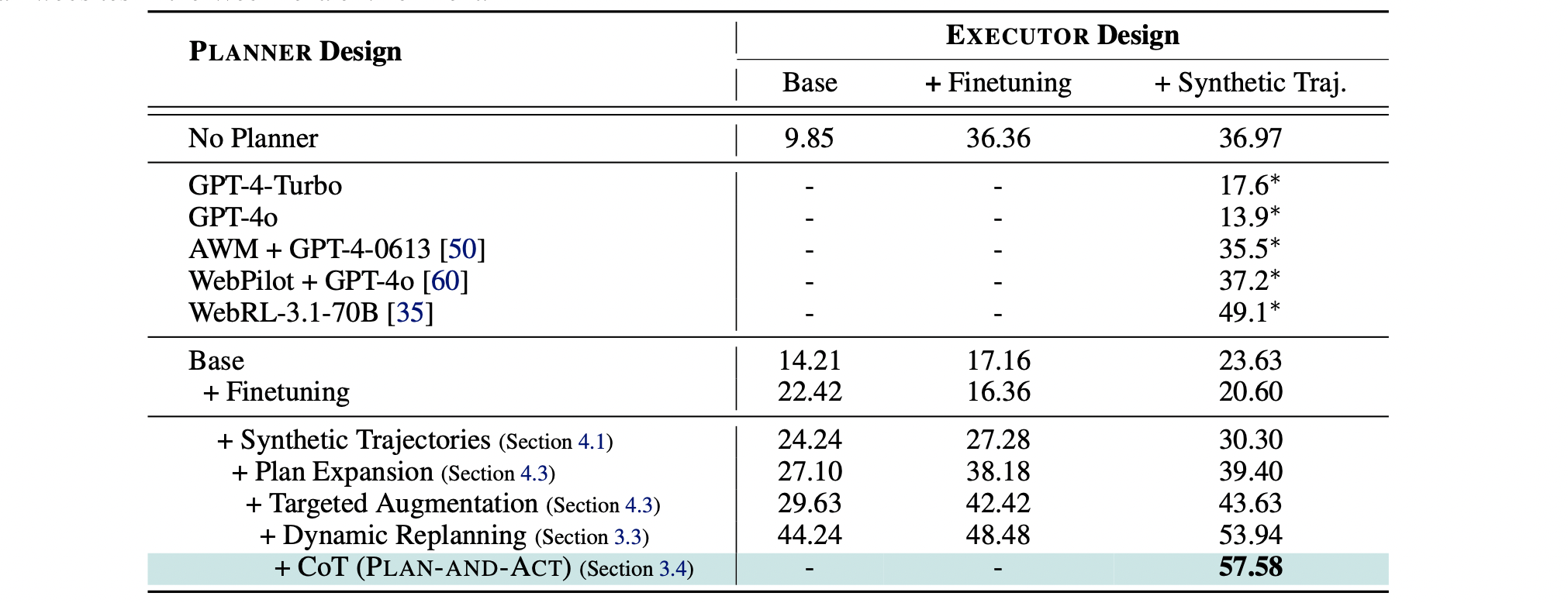
針對 Executor Design 部分:
- Base: 沒有經過 Fine-Tune 的 LLaMA-3.3-70B-Instruct
- +Finetuning: LLaMA-3.3-70B-Instruct 訓練在 WebArena-lite Environment 所提供的 1113 個訓練樣本
- +Synthetic Traj.: LLaMA-3.3-70B-Instruct 訓練在 WebArena-lite Environment 所提供的 1113 個訓練樣本 + 923 個合成樣本
從 No Planner 的結果可以發現到,即是對 Executor 進行訓練,或是增加 Executor 的訓練樣本,表現仍然不好。說明了 Planner 存在的必要性。
加入了 Base Planner (LLaMA-3.3-70B-Instruct) 後,確實在 Executor 未經過訓練的情況下可以提升整體表現 (9.85 到 14.21),但是卻很神奇的在 Executor 經過訓練的情況下表現不好 (EX. 從 36.36 變為 17.16 以及從 36.97 變為 23.63)。這個現象主要是因為經過 Finetune 的 Executor 已經與未經過的 Finetune 的 Planner 不對齊。換句話說,此時的 Executor 更偏好特定形式的 Plan 而不是原始的 Plan。
有趣的是,將 Base Planner + Finetuning 後,整體的表現也沒有改善太多。作者認為這是因為 Planner 只有 Finetune 在 WebArena-lite Environment 所提供的 1113 個訓練樣本,造成了 Overfitting 現象。
最後,將 Planner 訓練在 Synthetic Data 上,並加入 Dynamic Replanning 以及 CoT Reasoning 後,整體的表現大幅提升。
7 結語
本篇文章介紹了 PLAN-AND-ACT: Improving Planning of Agents for Long-Horizon Tasks 論文,理解如何針對 LLM Planning 的任務,設計一個 Planner 以及 Executor 合作的框架。更重要的是,如何透過一個 Synthetic Data Generation 的 Pipeline 分別產生 Planner 以及 Executor 的訓練資料,並且針對兩者加以訓練,更進一步提升表現。